Introduction
Imagine a world where machines not only understand your words but also grasp the nuances of human emotion, humor, and creativity. This isn't the premise of a futuristic sci-fi novel—it's the reality of large language models (LLMs) that are revolutionizing the way we interact with technology. In a staggering feat of engineering, these models can generate text that is not only coherent but often indistinguishable from that written by humans. But how do these digital marvels work? What lies behind the algorithms and data that empower them to converse, write, and even think in ways that mimic our own? Join us as we unravel the intricate tapestry of artificial intelligence, exploring the mechanics and magic of large language models that are shaping our digital landscape. Prepare to be amazed by the science that turns numbers and codes into the eloquent prose of the future.
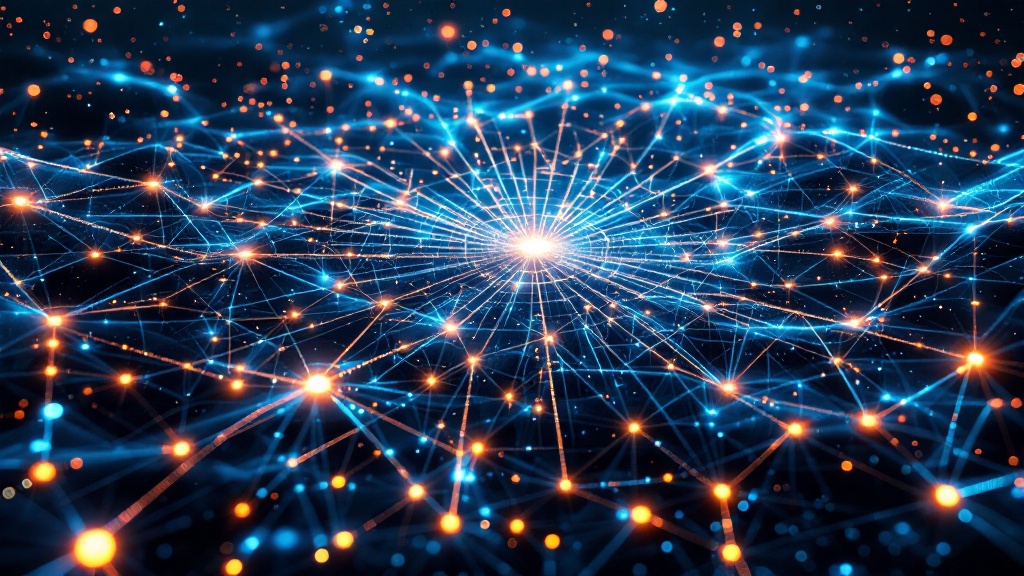
Understanding the Architecture of Large Language Models
Large language models (LLMs) are underpinned by transformer architecture, which empowers them to process and generate text that closely resembles human language. Central to their functionality is the self-attention mechanism, allowing these models to focus on relevant parts of input data while disregarding less pertinent information. During their training phase, LLMs engage in unsupervised learning across diverse datasets, a process that equips them with the ability to grasp context and nuance effectively. To enhance their performance in specific applications, such as chatbots, translation services, and content generation, fine-tuning on targeted tasks is crucial. However, as LLMs scale up with increased parameters and larger datasets, their performance improves significantly. This scalability, while beneficial, also brings forth concerns regarding resource consumption and the ethical implications of deploying such powerful technologies in society.
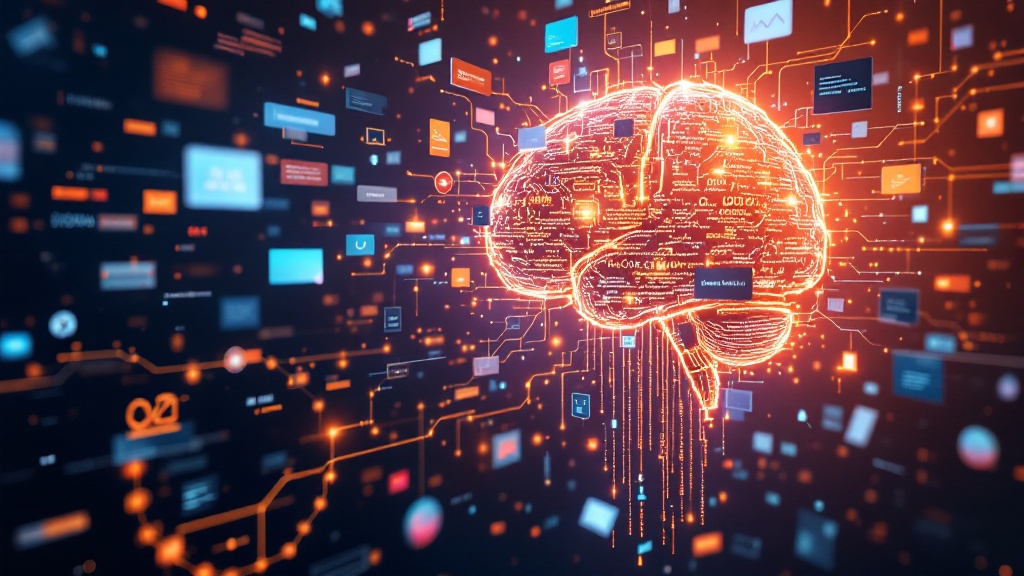
How Data Shapes Language Understanding?
Data serves as the foundation for training large language models, offering essential examples that enable them to learn various linguistic patterns. Without high-quality and diverse training data, a model's ability to grasp context and nuance would be severely limited. Iterative training plays a pivotal role in this process, as models adjust their parameters based on the feedback received from the data, thereby enhancing their predictive capabilities in a range of language tasks. Additionally, the continuous influx of new data is crucial; it allows these models to adapt and refine their understanding of evolving language use and trends. As a result, LLMs become more adept at handling real-world language challenges, ultimately making them more effective tools for communication and information retrieval.
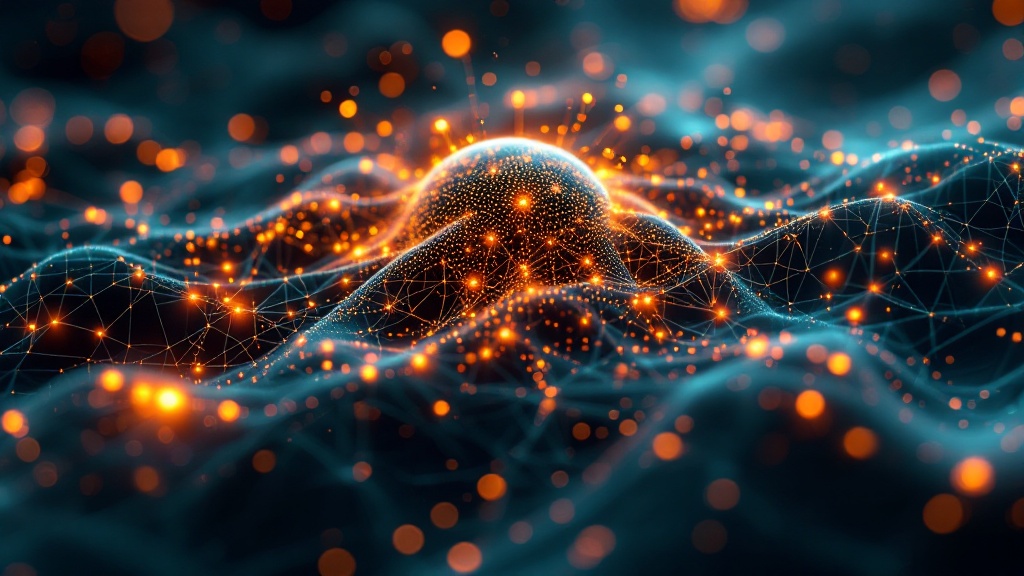
Exploring the Role of Neural Networks in Language Models
Neural networks play a crucial role in enhancing the capabilities of language models, as they empower these systems to learn intricate patterns embedded within text data. By utilizing sophisticated mechanisms like attention, these networks allow models to evaluate the relevance of each word in a given sentence, significantly improving contextual understanding. The advent of deep neural network architectures, particularly transformers, has revolutionized the way language models generate responses that are both coherent and contextually appropriate. As a result of training language models on vast datasets using these advanced neural networks, substantial advancements have been observed in various language tasks, including translation, summarization, and sentiment analysis. This evolution not only showcases the power of neural networks but also underscores their importance in shaping the future of natural language processing.
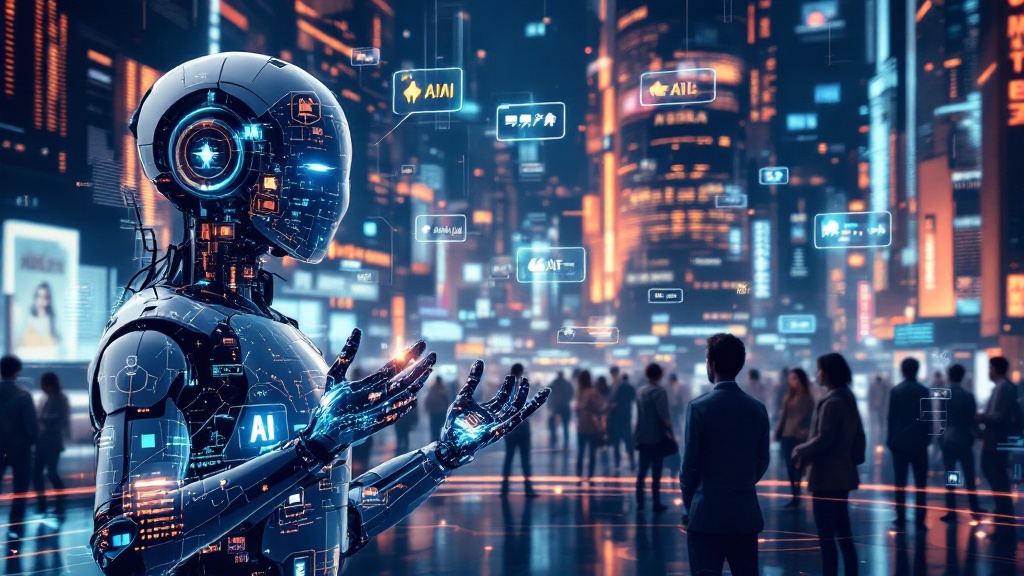
Applications of Large Language Models in Various Industries
The versatility of large language models (LLMs) is evident across various industries, significantly transforming how businesses operate. In customer service, these models enhance user experience by powering chatbots that analyze customer inquiries and complains which will deliver instant responses and tailored assistance, ensuring that clients receive help promptly. Similarly, the healthcare sector leverages LLMs to analyze patient medical records and generate comprehensive medical reports, aiding in accurate disease diagnosis. Financial institutions also benefit from these models, as they analyze market trends and automate trading strategies by processing extensive amounts of financial news and data, improving investment strategies. Furthermore, the education sector embraces LLMs to create personalized learning experiences, develop tutoring systems, and generate educational and training materials, ultimately fostering a more engaging and effective learning environment. Through these applications, large language models demonstrate their potential to revolutionize communication and decision-making across diverse fields.
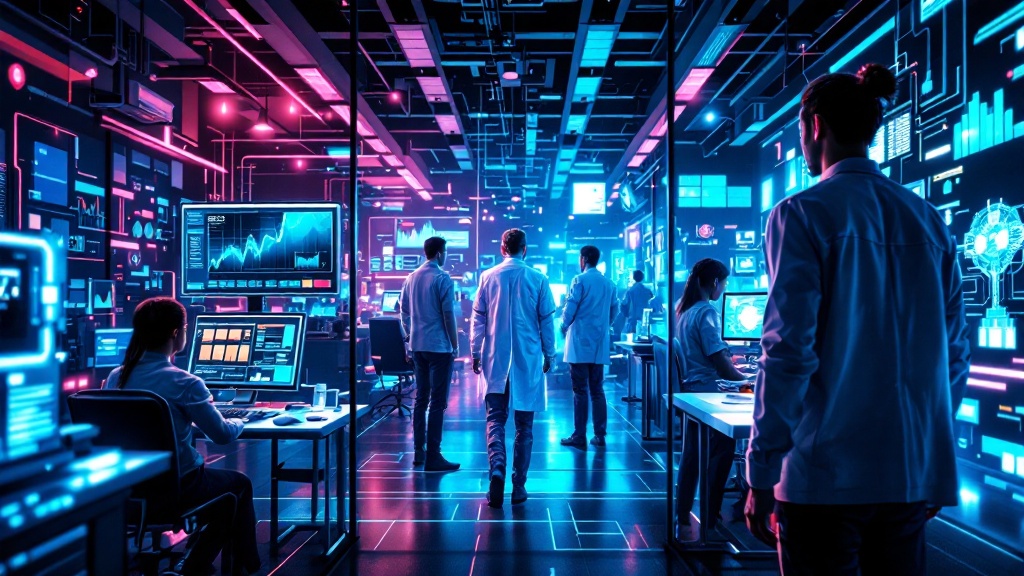
Future Trends in the Development of Language Models
Future advancements in large language models are poised to revolutionize their capabilities, particularly in multilingual understanding. With improved fluency across numerous languages, these models will break down communication barriers, enabling global interactions. Additionally, a greater focus on contextual understanding will empower them to generate nuanced responses, capturing subtleties in tone and intent that are often critical in human conversation. Ethical AI considerations will play a significant role in shaping development, driving efforts to reduce bias and promote responsible usage across various applications. As these technologies evolve, the collaboration between AI and human creativity will redefine content creation; language models will emerge as vital tools in writing, art, and communication, enhancing the creative process while offering innovative solutions to complex challenges.
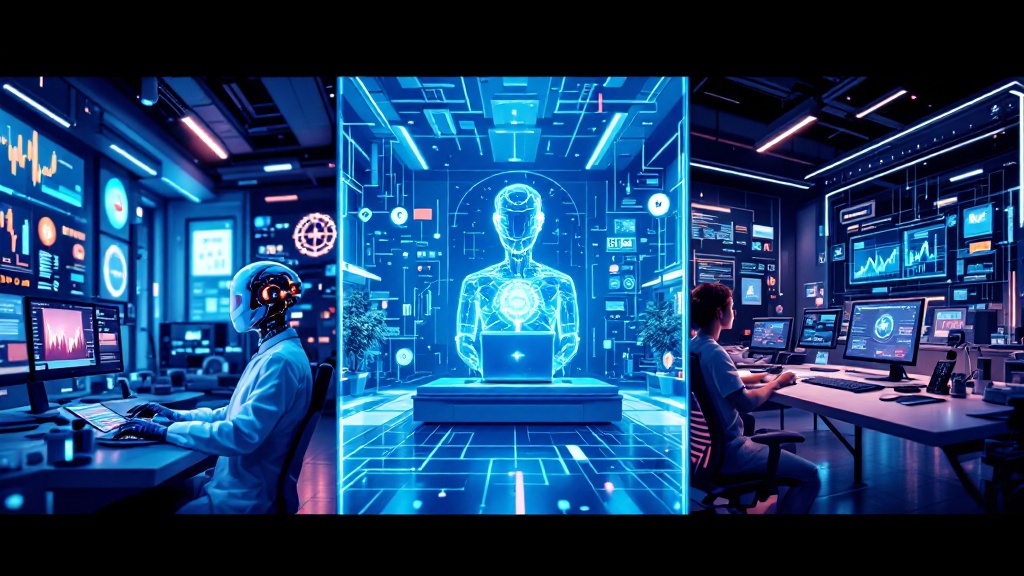
Conclusion
In conclusion, large language models (LLMs) represent a remarkable intersection of advanced technology and human language understanding, driven by intricate architectures and vast datasets. Their transformer-based architecture, equipped with self-attention mechanisms, allows them to process and generate text that closely mirrors human communication. The training process, grounded in high-quality data and iterative learning, equips these models with the contextual awareness necessary to tackle a wide array of language tasks. As industries increasingly adopt LLMs, their applications are transforming customer service, healthcare, finance, and education, enhancing efficiency and user experience while fostering innovation.