Introduction
Artificial Intelligence (AI) has rapidly evolved, particularly in the realm of text generation. From chatbots to content creation, AI models are now capable of producing human-like text with impressive fluency and contextual awareness. These advancements stem from a combination of Natural Language Processing (NLP), deep learning, and neural networks that allow machines to understand, interpret, and generate text in a way that closely resembles human communication.
At the heart of AI text generation lies sophisticated training processes that expose models to vast amounts of textual data. This enables them to learn grammar, sentence structure, and contextual relationships, allowing for more coherent and relevant output. By leveraging powerful architectures such as transformer-based models, AI can generate high-quality text that adapts to different tones and styles.
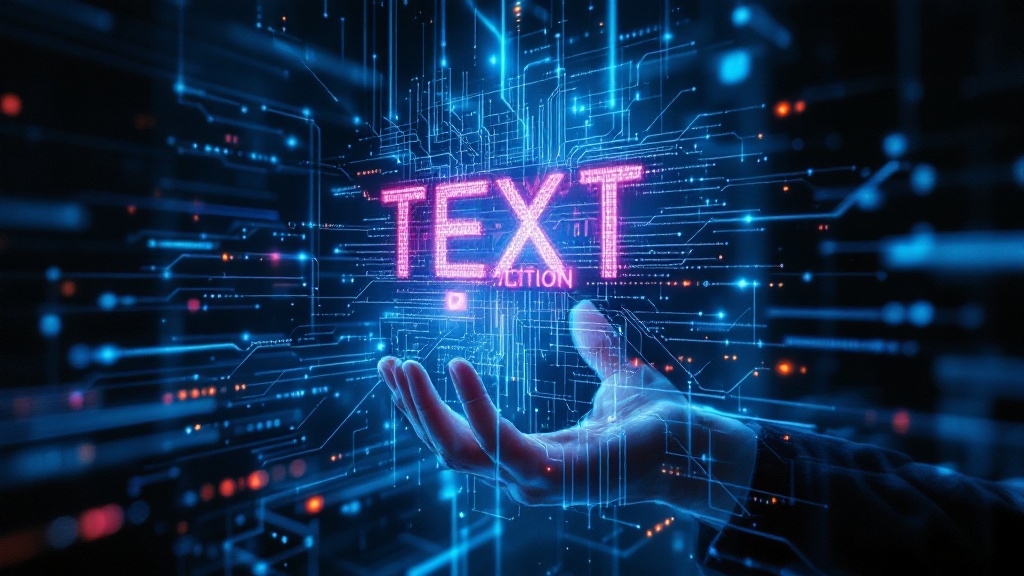
How AI Understands and Processes Language
AI text generation begins with Natural Language Processing (NLP), a field of AI that enables machines to interpret, analyze, and generate human language. NLP combines computational linguistics with machine learning to process text in a way that mimics human understanding.
The process starts with breaking down sentences into smaller components, known as tokenization. These tokens are then assigned numerical representations called word embeddings, allowing AI to recognize patterns and relationships between words. AI models further refine this understanding through context-aware processing, ensuring that words are interpreted correctly based on their surrounding text.
Additionally, NLP models use syntactic and semantic analysis to understand grammar rules and meanings behind words. Sentiment analysis and named entity recognition further enhance the model’s ability to process human language, making AI-generated text more coherent and relevant to user queries.
To appreciate the depth of AI-generated text, it helps to understand how large language models function at their core, as explained in How Does Large Language Models Work.
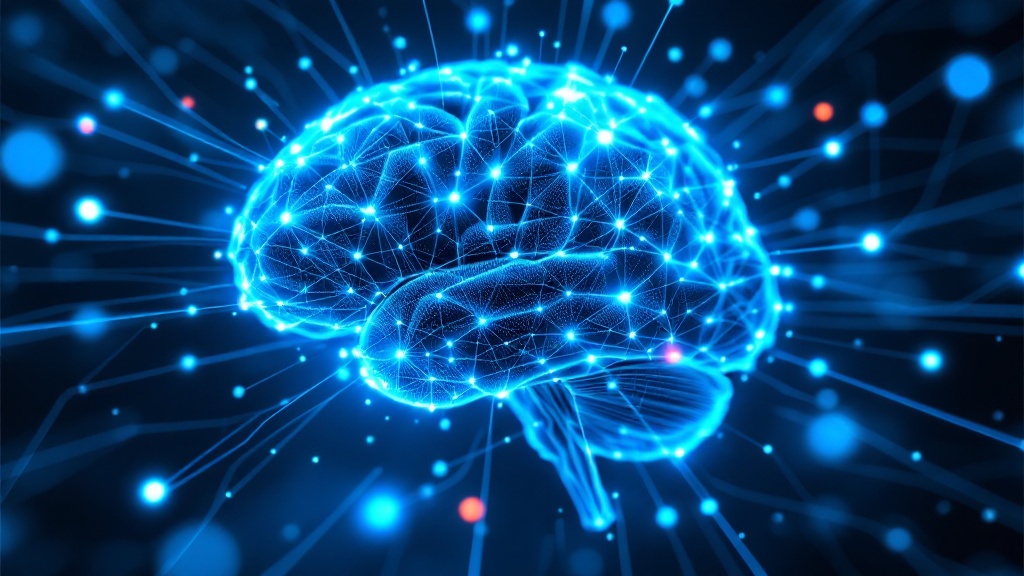
The Role of Neural Networks in AI Text Generation
At the core of AI text generation lies deep learning, particularly neural networks. These networks, inspired by the human brain, are designed to process vast amounts of text data and learn from it. Transformer-based architectures, such as those found in modern language models, leverage self-attention mechanisms to capture contextual relationships between words, enabling AI to produce coherent and contextually relevant text.
Transformers, like those used in Generative Pre-trained Transformer (GPT) models, use multiple layers of attention mechanisms to weigh the importance of words in a sentence. This allows the AI to understand nuances, idiomatic expressions, and even stylistic elements of writing. By continuously analyzing new data and refining their algorithms, neural networks improve their ability to generate text that aligns with human-like language fluency.
The growing sophistication in AI-generated content is fueled by innovations in context-aware and emotionally intelligent systems, as highlighted in AI is Becoming More Context-Aware and Emotionally Intelligent.
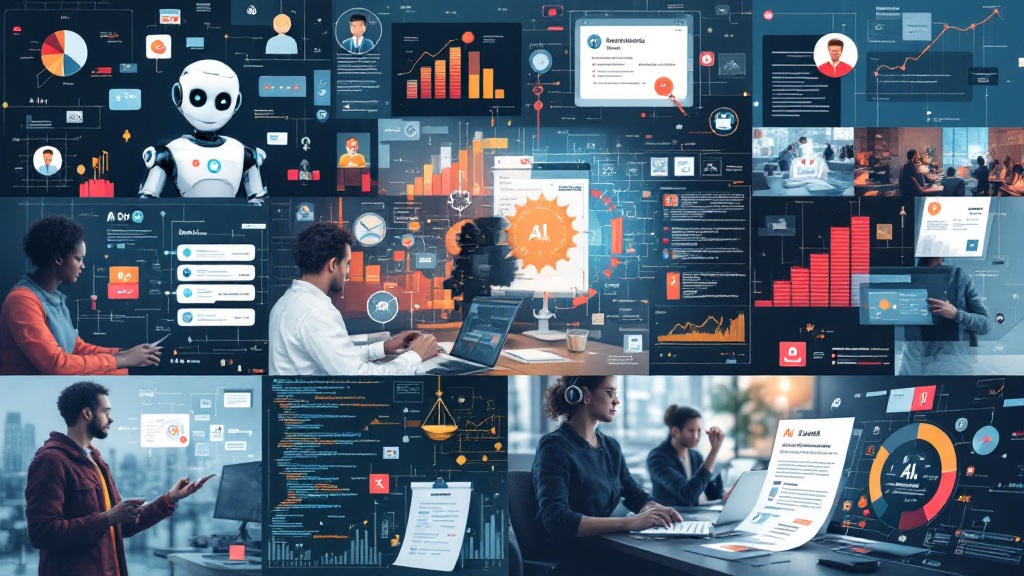
Training AI to Generate Text: The Learning Process
AI text generation models undergo extensive training on massive datasets to refine their ability to understand and produce language. This training process typically consists of:
- Pretraining: The model is exposed to large text corpora to learn linguistic structures, grammar, and contextual relationships. During this phase, AI learns word associations, sentence structures, and conversational patterns.
- Fine-tuning: The pretrained model is further refined on specific datasets or tasks to improve its accuracy in particular applications. For example, an AI model designed for medical applications will be fine-tuned using scientific literature and clinical notes to ensure its responses align with medical terminology and best practices.
- Reinforcement Learning: Some models incorporate feedback loops where human reviewers help refine AI-generated responses for better alignment with human expectations. Through reinforcement learning with human feedback (RLHF), AI improves its ability to generate appropriate, useful, and unbiased content.
- Self-Supervised Learning: AI models also leverage self-supervised learning techniques, where they predict missing words in sentences or reassemble shuffled text. This helps AI gain a deeper understanding of how words and phrases function within context.
Many AI-generated text tools today rely on retrieval-augmented generation (RAG) to provide more accurate, informative outputs—covered in What is Retrieval-Augmented Generation (RAG) Explained.
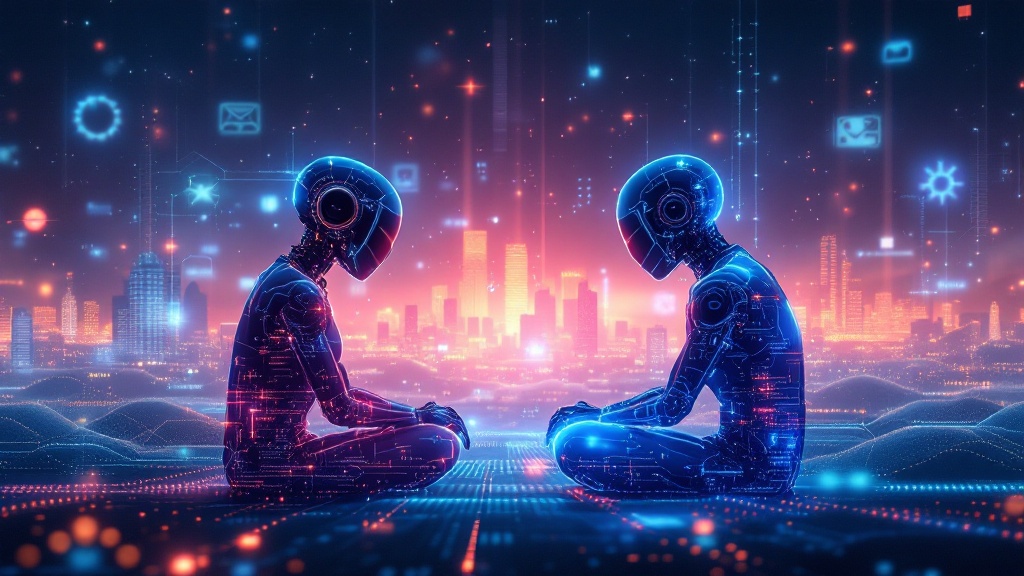
Real-World Applications of AI-Generated Text
The versatility of AI text generation is evident in various industries, revolutionizing multiple domains:
- Automated Content Creation: AI assists writers in generating blog posts, reports, and marketing copy efficiently. Businesses leverage AI to produce high-quality content in less time, improving productivity while maintaining creativity.
- Conversational AI: Virtual assistants and chatbots use AI text generation to deliver real-time, context-aware responses. This improves customer service efficiency and enhances user experience.
- Code Generation: AI models help developers by generating code snippets and debugging suggestions. This accelerates software development by reducing manual coding efforts.
- Personalized Learning: AI-powered tutors create adaptive learning materials tailored to individual student needs, offering custom explanations and exercises based on learner progress.
- Legal and Compliance Documentation: AI can draft contracts, summarize case laws, and assist in regulatory compliance by generating structured legal documents.
As AI tools evolve, they are playing a major role in boosting productivity for writers and marketers, something discussed in Boosting Productivity with AI-Powered Writing Assistants.
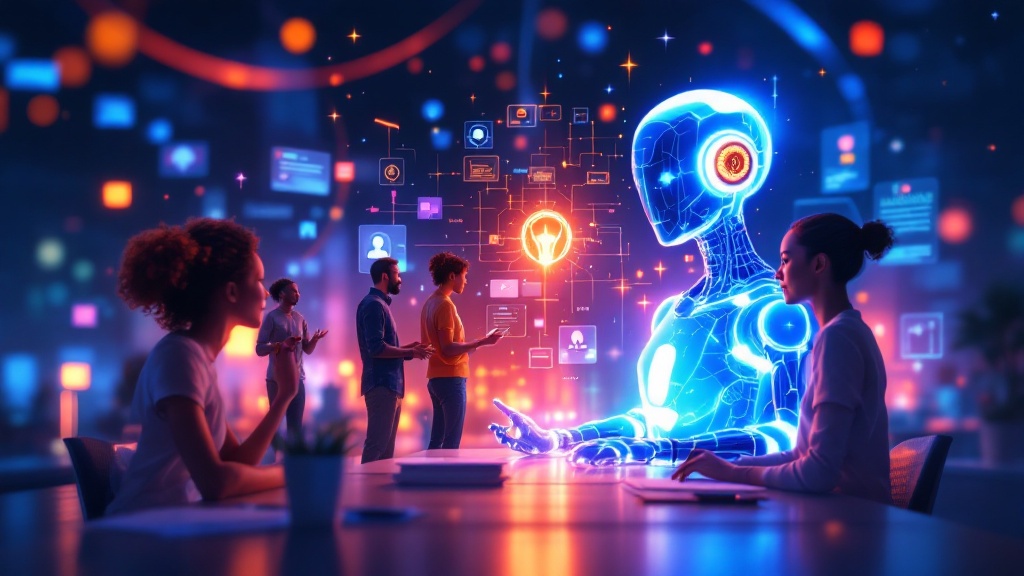
Ethical Considerations and Challenges
Despite its advancements, AI text generation presents challenges, particularly in terms of bias, misinformation, and ethical concerns. Some key issues include:
- Bias in Training Data: AI models can inherit biases present in their training data, leading to skewed or misleading outputs. This is especially concerning in fields like hiring, law enforcement, and healthcare, where biased AI-generated content can have serious consequences.
- Misinformation Generation: AI can inadvertently produce false or misleading content, raising concerns about trust and reliability. It is crucial to ensure fact-checking mechanisms are in place before AI-generated content is used for critical decision-making.
- Content Authenticity: The ability of AI to generate human-like text blurs the line between human and machine-generated content, necessitating transparency and regulation. Watermarking and AI-generated content disclosure policies can help address this issue.
- Data Privacy Risks: AI models that generate text may sometimes inadvertently include sensitive or personal data extracted from their training sets. Developers must implement privacy-focused AI training techniques to mitigate risks.
The science behind AI text generation is also changing how we interact with brands through smart customer support systems, like those featured in AI-Powered Chatbots Are Revolutionizing Customer Support.
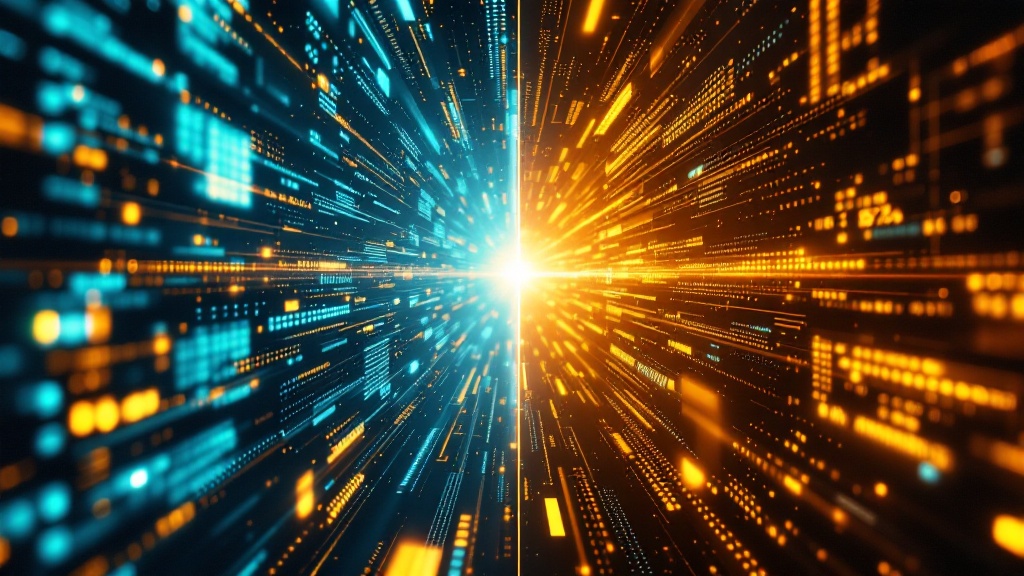
Conclusion
In conclusion, AI text generation is a testament to the power of machine learning and neural networks in shaping the future of communication. By leveraging NLP, deep learning, and advanced training techniques, AI has transformed the way we interact with language. While challenges remain, the potential for AI-driven text generation to enhance creativity, efficiency, and accessibility is undeniable. As research and innovation progress, AI-generated text will continue to play a pivotal role in various fields, driving the next wave of digital transformation.
The future of AI text generation holds exciting possibilities, with advancements that promise to make AI even more intelligent, reliable, and useful. As we embrace this evolving technology, balancing innovation with ethical considerations will be key to harnessing the full potential of AI in text generation. Visit our blogs page now.
Leave a Reply