Introduction
The evolution of artificial intelligence necessitates structured methodologies to improve contextual understanding and interaction efficiency. The Model Context Protocol (MCP) serves as a framework for optimizing AI’s ability to process, retain, and apply context across multiple interactions. By integrating structured context management, AI systems can deliver more accurate, relevant, and coherent responses while enhancing user experience. Moreover, MCP reduces redundant information processing, allowing AI to focus on the most critical aspects of communication, ensuring smoother and more efficient interactions between humans and machines.
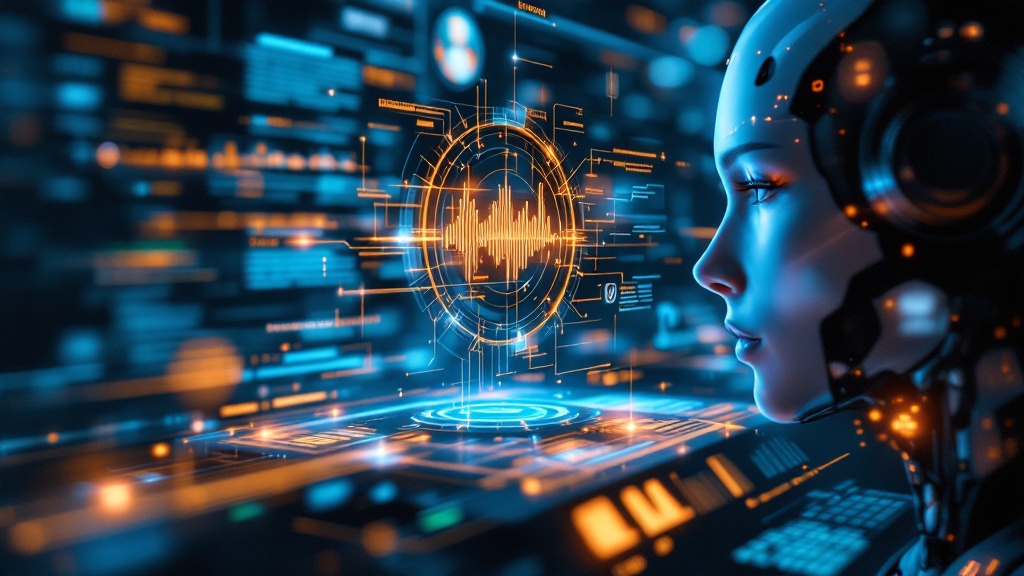
Understanding Model Context Protocol
MCP is a systematic approach to managing and structuring context within AI-driven systems. Traditional AI models process user input statically, often lacking continuity, personalization, and dynamic adaptation. MCP introduces structured mechanisms that allow AI to retain contextual information across extended interactions, prioritize relevant data while filtering unnecessary details, and dynamically adjust responses based on prior exchanges. Additionally, MCP enhances memory recall for more natural conversations, improves alignment with user preferences and objectives, and enables AI to adapt to new topics seamlessly by integrating prior knowledge efficiently. It also helps mitigate response inconsistencies by analyzing past interaction trends and streamlining dialogue structures to increase overall response clarity. By implementing MCP, AI systems can function with greater contextual awareness, reducing redundancy and increasing efficiency in interactions. This allows AI to mimic human-like conversational patterns, creating an intuitive and fluid user experience.
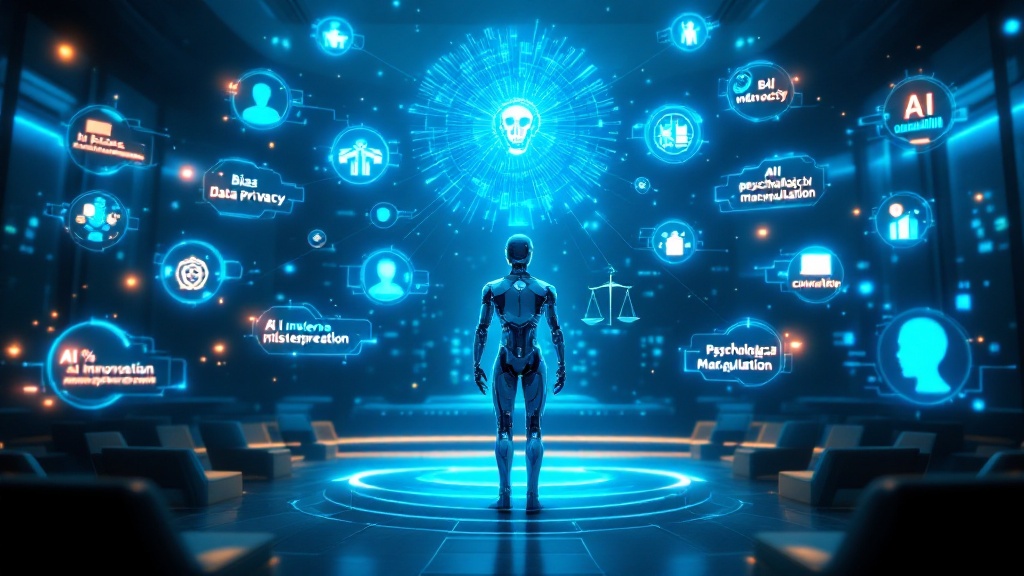
Key Components of Model Context Protocol
MCP consists of multiple core components that facilitate context management, adaptability, and relevance in AI models:
Context Retention: AI models store previous exchanges, ensuring continuity and logical progression in conversations.
Data Structuring: Hierarchical organization of context for efficient recall and accurate association with new data.
Memory Optimization: Filtering mechanisms that prioritize essential information and prevent information overload.
User Intent Recognition: Continuous adaptation based on user behavior, objectives, and interaction patterns.
Session-Based Context Control: Balancing short-term and long-term contextual memory to ensure relevance without excessive recall.
Cognitive Load Reduction: Automating context application to minimize the need for repetitive user input.
Personalization Algorithms: AI adjusts its tone, complexity, and engagement level based on user preferences.
Error Handling Mechanisms: Detecting and correcting misinterpretations within an ongoing conversation to ensure accuracy.
These components create a framework where AI can process and apply knowledge in a structured manner, enhancing interaction quality and reducing potential misunderstandings.
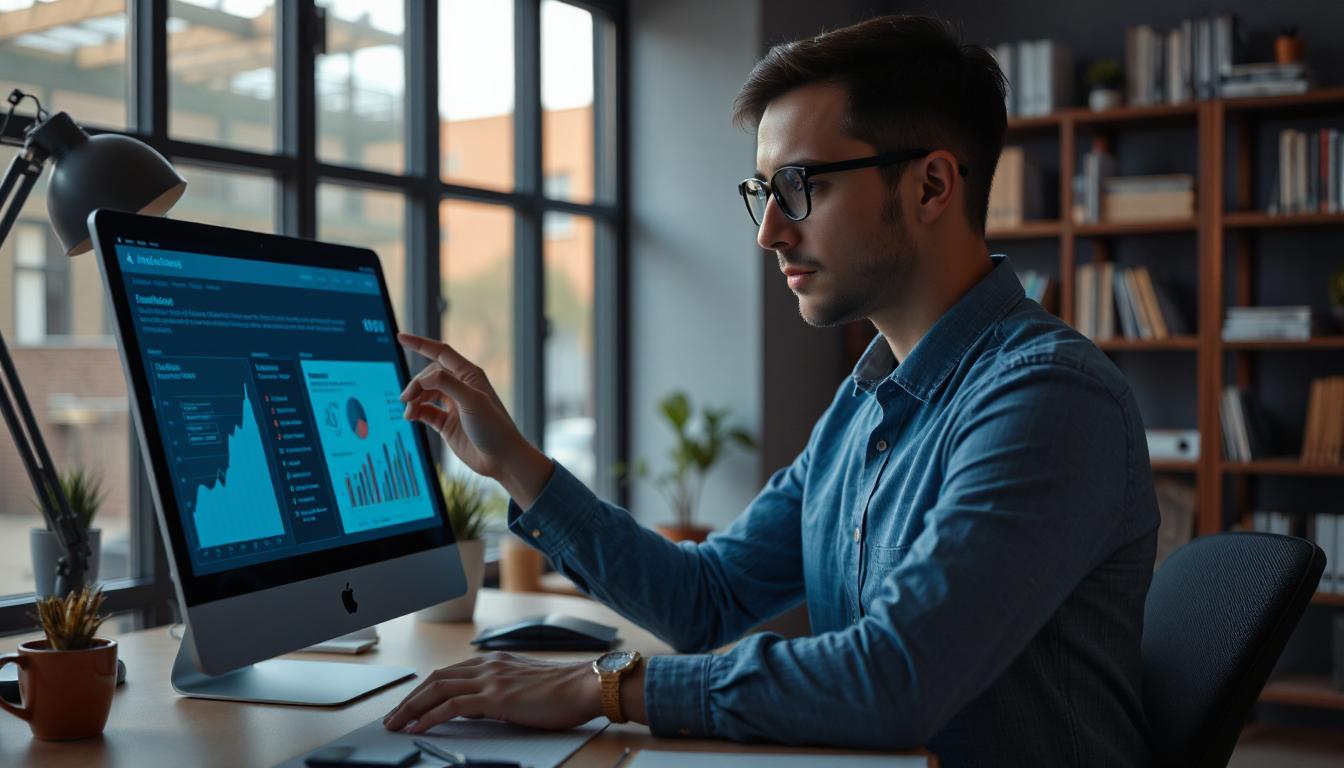
Applications of Model Context Protocol
MCP is highly adaptable across various industries, offering improvements in efficiency, personalization, and user engagement:
Customer Support: AI chatbots maintain conversation history for better resolution tracking, minimizing the need for users to repeat information.
Education: Personalized learning experiences through context-aware tutoring systems that adapt to each student’s progress and knowledge gaps.
Healthcare: AI retains patient history for precise diagnosis assistance, reducing errors in medical recommendations.
Content Creation: AI-generated content aligns with user preferences and past inputs, creating a consistent tone and messaging strategy.
Finance: AI-driven advisory tools can provide more precise investment recommendations based on user financial behaviors.
These are the common applications that demonstrate MCP’s ability to refine AI-driven solutions, ensuring more intuitive and effective interactions. As MCP continues to develop, it will expand its capabilities, making AI systems more adaptable across diverse fields.
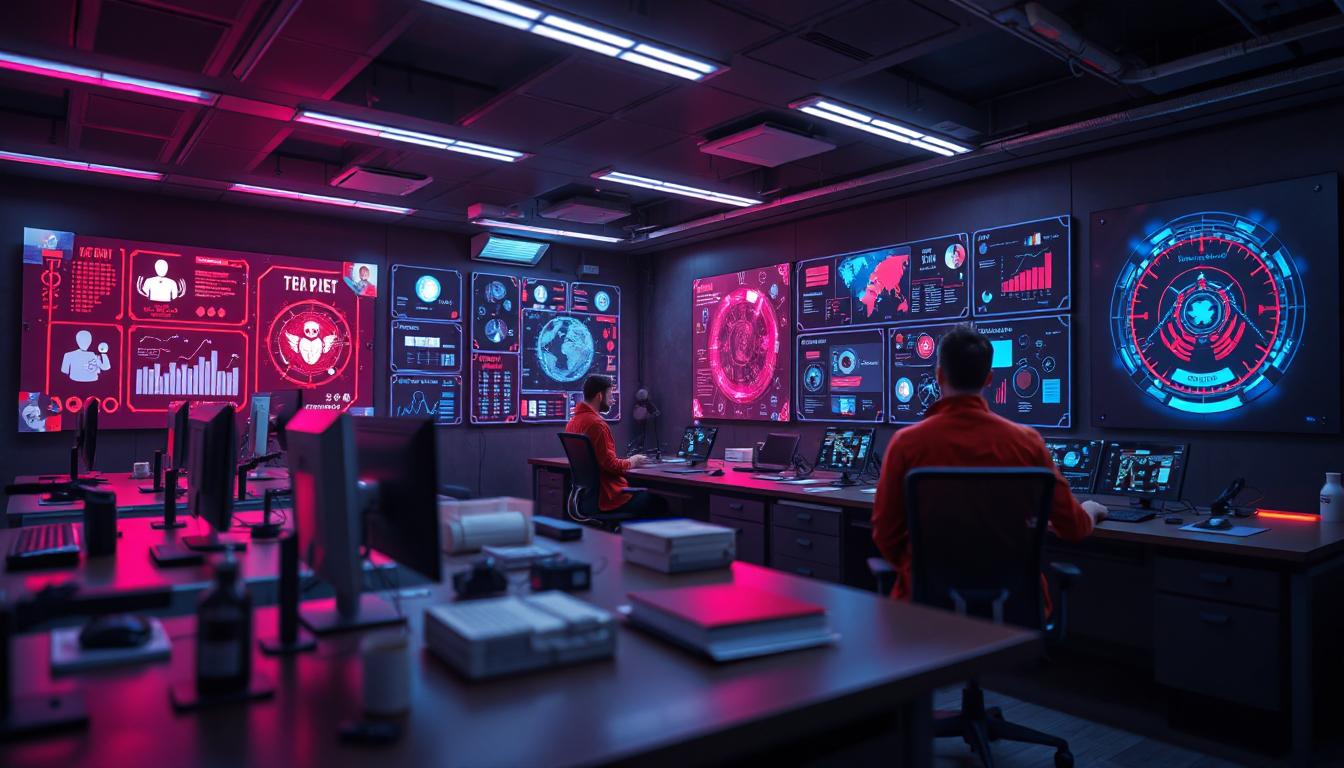
The Role of Model Context Protocol in Human-AI Collaboration
As Artificial Intelligent systems become more sophisticated, human-AI collaboration is evolving to become more seamless and effective. MCP plays a crucial role in facilitating this collaboration by ensuring that AI can understand, remember, and appropriately apply contextual information. AI models equipped with MCP can better support human decision-making by retaining critical insights from previous interactions, reducing repetitive explanations, and adapting responses to specific user needs. This allows professionals in various fields—such as research, engineering, and creative industries—to leverage AI as a reliable assistant. MCP-driven AI can serve as an extension of human cognition, enhancing productivity while maintaining a natural and intuitive workflow. By bridging the gap between AI-generated insights and human expertise, MCP strengthens the synergy between technology and its users, fostering more efficient problem-solving and innovation.
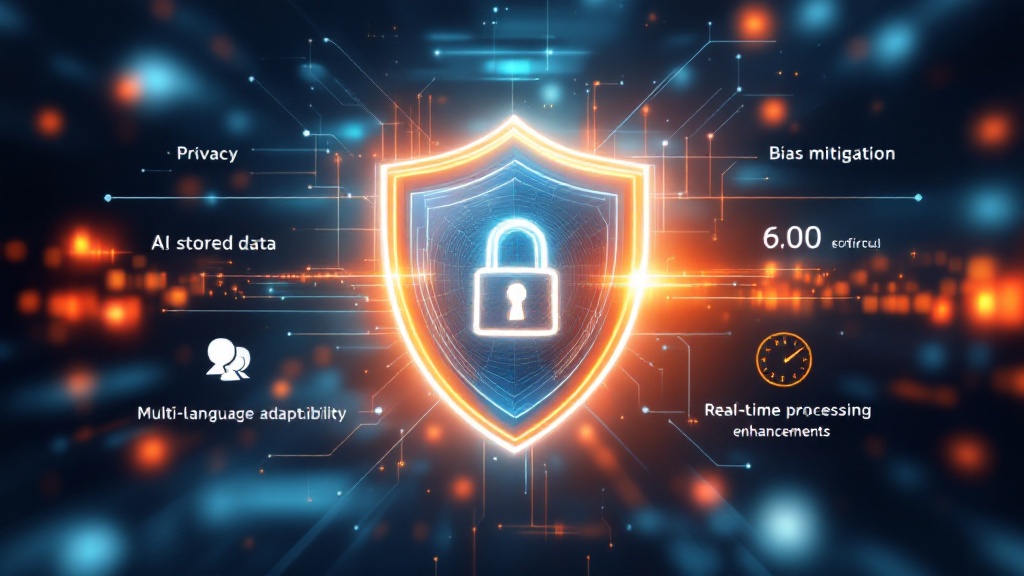
Challenges and Future Development of MCP
Despite its advantages, MCP faces challenges that must be addressed for optimal deployment:
Data Privacy and Security: Managing stored context without compromising confidentiality and ensuring compliance with data protection laws.
Scalability: Balancing computational efficiency while expanding contextual depth, particularly in large-scale enterprise AI deployments.
Bias Mitigation: Ensuring fair and neutral context interpretation by continuously auditing training data for hidden biases.
Adaptive Learning Models: Enhancing AI’s ability to evolve with user behavior, learning from interactions without requiring frequent manual retraining.
Cross-Platform Context Sharing: Standardizing MCP for seamless integration across different AI systems and platforms to provide consistent context management.
User-Controlled Context Management: Developing user-friendly tools that allow individuals to customize how AI retains and applies their contextual data.
Latency Reduction: Enhancing AI’s real-time processing capabilities to minimize delays in generating context-aware responses.
Multi-Language Adaptability: Ensuring MCP functions effectively across various linguistic structures, maintaining accuracy and coherence in translations.
As AI technology advances, continuous refinement of MCP will lead to smarter, more context-aware AI models capable of delivering seamless, intelligent interactions. The development of hybrid AI models that combine symbolic reasoning with deep learning approaches will further enhance MCP's capabilities, allowing AI to handle complex contextual queries more effectively.
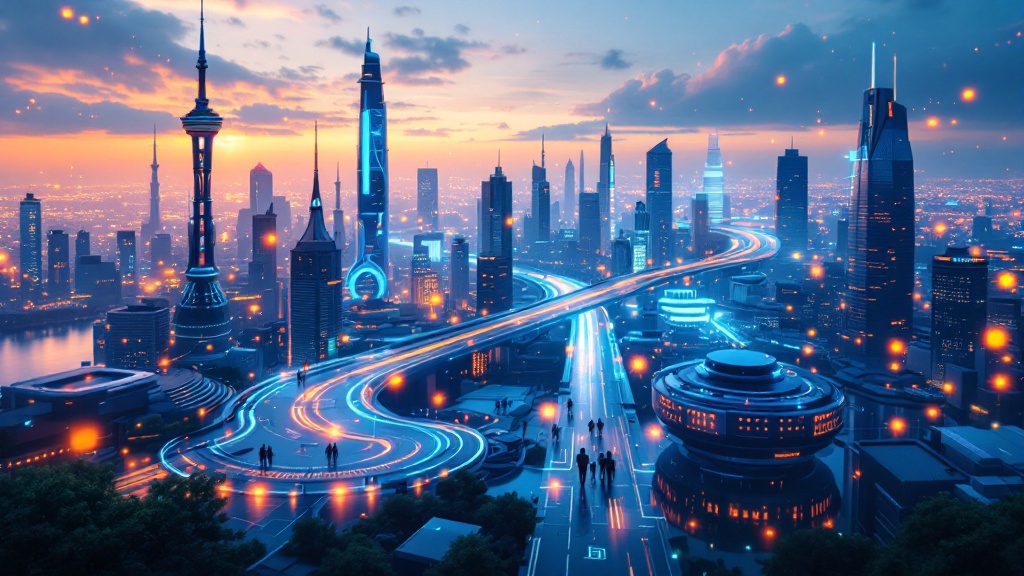
Conclusion
The Model Context Protocol is a crucial innovation in AI development, enabling systems to process, retain, and utilize context effectively. By integrating MCP, AI-driven solutions become more intuitive, responsive, and user-centric, leading to enhanced efficiency across industries. As AI continues to evolve, MCP will play a fundamental role in shaping the next generation of intelligent, context-aware technologies.
With organizations striving for AI-driven efficiency, MCP adoption ensures higher accuracy, better interaction quality, and improved user experience. The potential for MCP extends beyond current applications, paving the way for more advanced, adaptive AI models that can revolutionize digital communication, automate decision-making, and elevate the overall effectiveness of AI-powered systems.