Introduction
Imagine a world where machines not only recall vast amounts of information but also weave that knowledge into coherent, contextually rich narratives. Enter Retrieval-Augmented Generation (RAG), a groundbreaking approach in artificial intelligence that marries the precision of data retrieval with the creativity of generative models. This innovative fusion allows AI to not merely regurgitate facts but to craft responses that are informed, relevant, and surprisingly nuanced. If you’ve ever marveled at how quickly your virtual assistant can pull together information, you’ve witnessed the magic of RAG in action. In this exploration, we’ll delve into the mechanics of RAG, uncovering how it transforms the way we interact with information and reshapes the landscape of AI-driven communication.
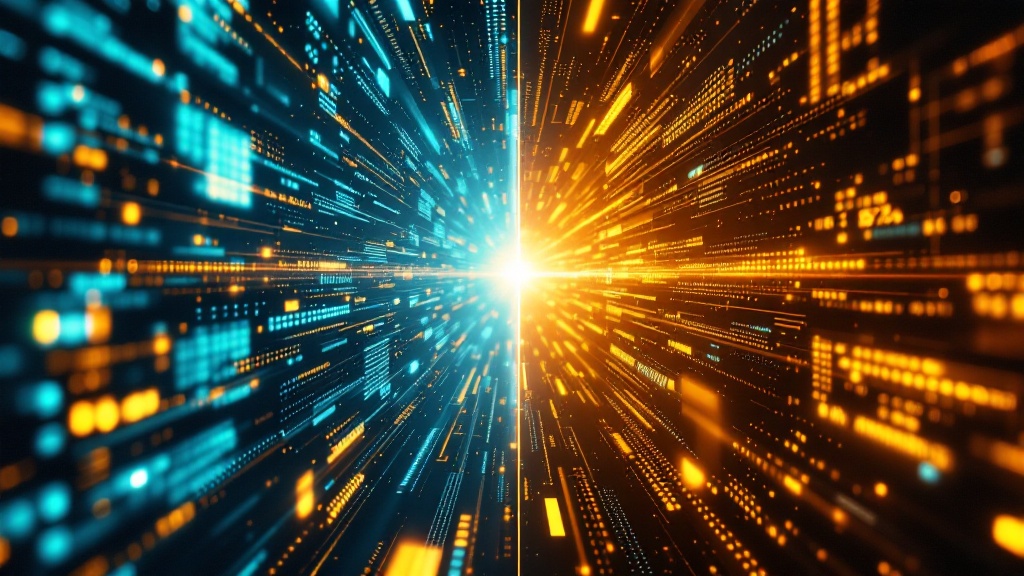
What is Retrieval-Augmented Generation?
Retrieval-Augmented Generation represents a significant advancement in the field of natural language processing by merging retrieval-based methods with generative models. This innovative approach allows RAG to tap into external knowledge sources, enhancing the accuracy and relevance of the information it provides. As a result, models utilizing RAG demonstrate improved performance in tasks that demand high levels of factual accuracy, such as answering questions and summarizing content. By integrating a retrieval mechanism within the generation process, RAG systems can generate outputs that are not only more informative but also more contextually relevant compared to traditional generative models. Ultimately, this synthesis of retrieval and generation paves the way for more sophisticated applications in various domains, ensuring that users receive responses that are both accurate and meaningful.
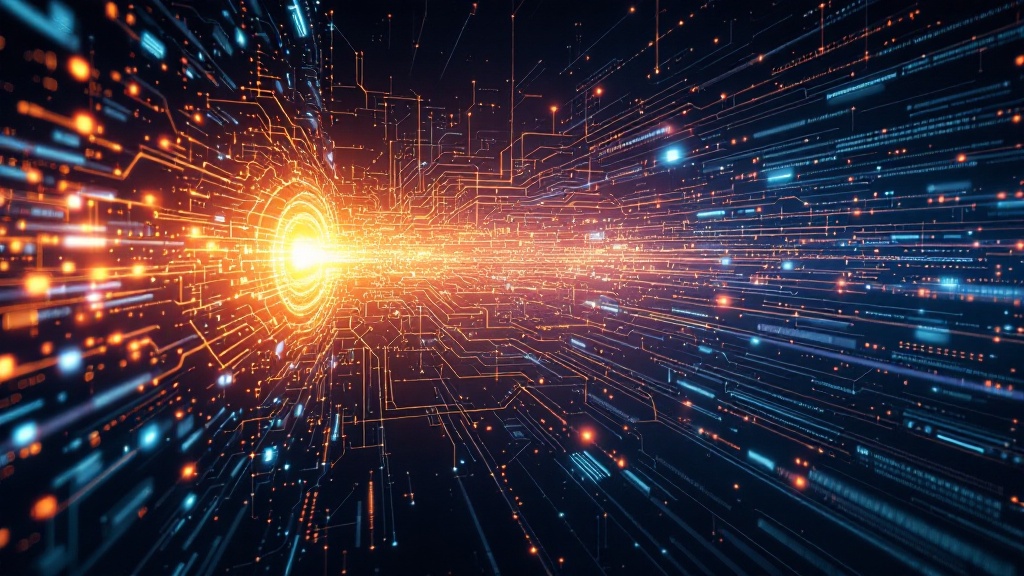
How does Retrieval-Augmented Generation work?
Combining Retrieval and Generation: Retrieval-Augmented Generation uniquely merges information retrieval with text generation, which significantly enhances the accuracy and relevance of the responses it produces. This combination allows RAG to utilize both existing knowledge and generative capabilities.
Document Retrieval Process: In the RAG framework, the initial step involves retrieving pertinent documents from a vast knowledge base. This retrieval process ensures that the generated text is informed by relevant information, leading to more accurate and contextually appropriate outputs.
Encoding and Searching: The method begins with encoding the input query, which is then used to perform a search across an extensive corpus of documents. This systematic approach allows for the identification of the most relevant information before generating a response.
Dynamic Knowledge Integration: By leveraging pre-trained language models along with retrieval mechanisms, RAG dynamically incorporates external knowledge into its responses. This capability markedly improves performance, especially for tasks that require a high degree of factual accuracy, ensuring that users receive well-informed and meaningful answers.
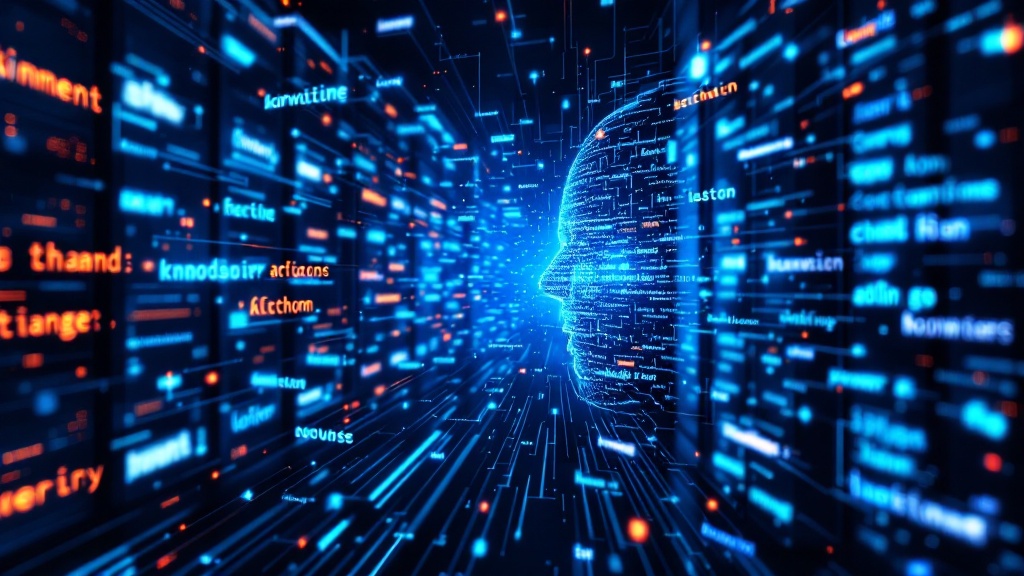
How RAG Enhances Natural Language Processing
Retrieval-Augmented Generation significantly enhances natural language processing tasks by integrating retrieval-based mechanisms with generative models, thereby improving contextual relevance. By leveraging external knowledge sources, RAG is capable of delivering more accurate and informative responses to user queries, addressing the need for reliable information. Furthermore, the architecture of RAG enhances the efficiency of language models by dynamically retrieving pertinent information, which reduces the reliance on extensive training data. This unique approach allows RAG to better handle long-term dependencies in text, resulting in outputs that are not only coherent but also contextually appropriate, ultimately enriching user interactions.
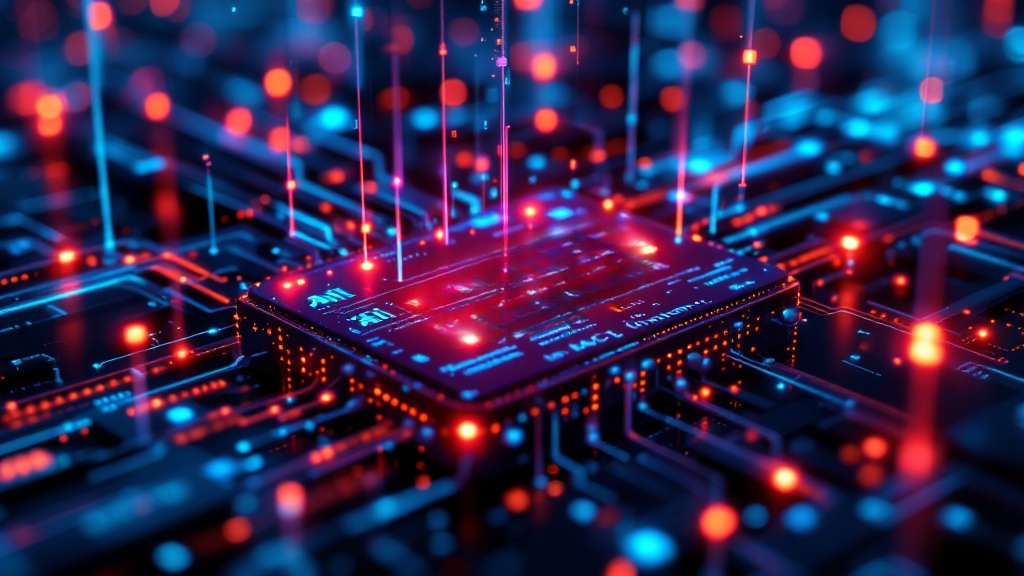
Applications of RAG in Modern AI Solutions
Retrieval-Augmented Generation transforms artificial intelligence applications by enhancing the accuracy and relevance of responses through the integration of external data sources. This approach provides contextually rich information, improving user experiences in chatbots and virtual assistants that access extensive knowledge databases in real-time. RAG models excel in natural language processing tasks like summarization and question answering, delivering precise results. Additionally, content creation tools utilize RAG to help writers generate ideas and drafts by synthesizing diverse textual sources, promoting creativity and coherence.
Enhanced Accuracy: RAG integrates external data sources to provide contextually relevant information, leading to more precise responses. This is particularly valuable in AI-driven research tools, where accurate data retrieval enhances decision-making and analysis.
Improved Conversational Quality: In chatbots and virtual assistants, RAG accesses vast databases of knowledge in real-time, resulting in more engaging interactions. These AI-powered systems are commonly used in customer support, virtual tutoring, and interactive FAQ solutions, ensuring users receive well-informed responses.
Effective Information Retrieval: RAG models facilitate better information retrieval in natural language processing tasks, yielding accurate results in summarization and question answering. AI-driven document analysis tools leverage this capability to extract key insights from large datasets, making them essential for industries dealing with vast amounts of textual information.
Support for Content Creation: By pulling from diverse textual sources, RAG helps writers generate ideas and drafts, ensuring creativity and coherence in their content. This feature is widely applied in AI-powered writing assistants, automated content generators, and editorial support tools, streamlining the content development process while maintaining originality and quality.
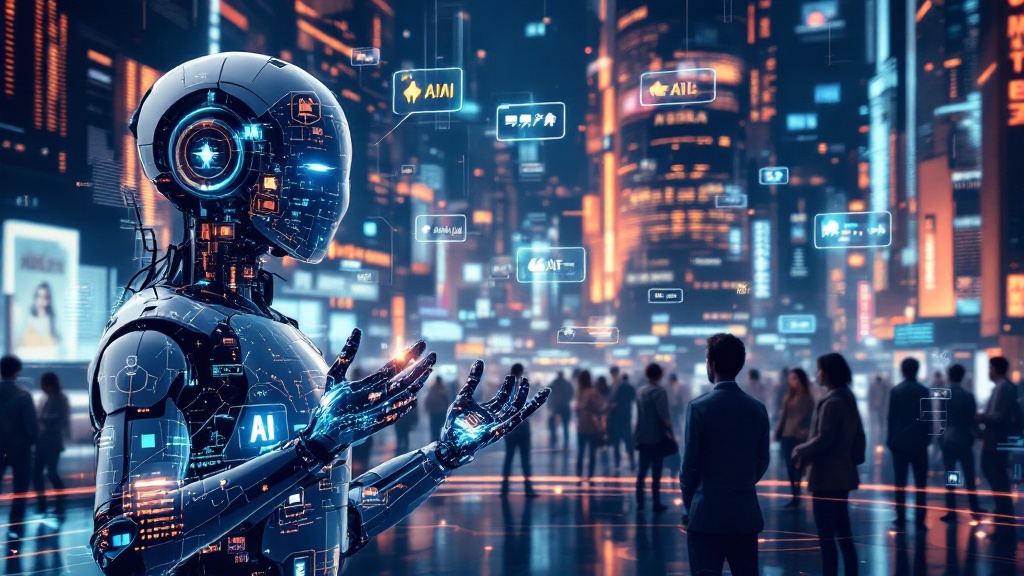
Comparing RAG with Traditional Text Generation Models
Retrieval-Augmented Generation represents a notable evolution in text generation by integrating external knowledge retrieval with generative models. This innovative approach significantly enhances the accuracy and contextual relevance of the generated text, enabling RAG to provide timely and specific information that traditional models often struggle to deliver. Traditional text generation models, on the other hand, rely solely on learned patterns from their training data, which can result in outdated or generalized outputs. In contrast, RAG dynamically pulls in relevant data from a knowledge base during the generation process, ensuring that the responses are informed by current knowledge. Although this retrieval step may demand more computational resources, potentially impacting efficiency, the trade-off is often justified by the richer and more accurate information that RAG can produce compared to its simpler, speed-focused counterparts.
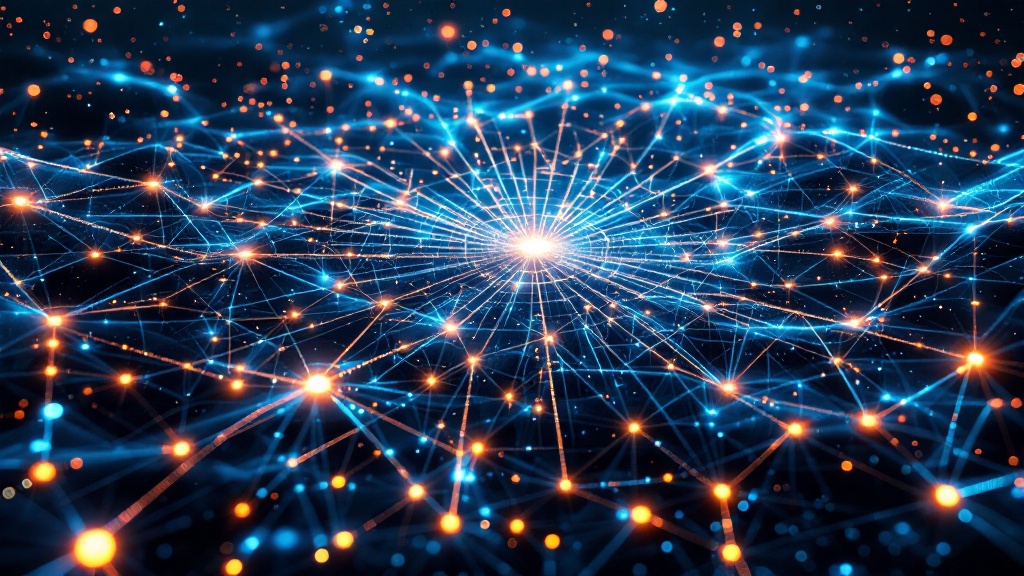
Conclusion
In conclusion, Retrieval-Augmented Generation (RAG) has emerged as a groundbreaking approach in natural language processing, marrying the strengths of information retrieval with the capabilities of generative models. By seamlessly integrating external knowledge sources, RAG enhances the accuracy, relevance, and contextual appropriateness of the outputs it generates. This innovative framework allows for real-time access to up-to-date information, leading to more meaningful user interactions and improved performance across various applications—from chatbots to content creation tools. As RAG continues to evolve, its capacity to refine prompts and dynamically refresh external data positions it as a vital asset in the quest for more sophisticated and reliable AI solutions. As we move further into an era where meaningful and accurate information is paramount, RAG stands out as a pivotal player, ensuring that users receive responses that are not only coherent but also grounded in the latest insights and knowledge. You can check out our other bogs about Large Language Models and Generative Pre-Trained Transformer.